ABSTARCT
Over a period of time one of the consequences of diabetes could be damage to the retina of the eye causing problems with eyesight the condition is as known as diabetic retinopathy. It is common complication of diabetes. To understand diabetic retinopathy let’s have look in the below image. The part of the of the front of the eye is called the cornea, the light passes through the cornea and hits the lens, the lens focuses the light on the back of the eye onto a light-sensitive lining called a retina, before exiting the back of the eye through the optic nerve there are blood vessels that travel through the retina and exit the back of the eye through the middle of the optic nerve. Over time uncontrolled high sugar levels can affect this blood vessels as a result, they might leak blood or any other fluids causing swelling and damage to the retina. In the early stages of diabetic retinopathy there are no symptoms or very mild symptoms that may go unnoticed as diabetic retinopathy progresses the following signs and symptoms may occur. Floaters on your filed of vision. Floaters are tiny dark specks or strings that move around the rear chamber of the eye causing you to see spots. This kind of difficulty faced same as night. Pain or pressure in one eye or both eyes. Usually both eyes get affected by diabetic retinopathy. Through the survey approximately 1 out of 10 adults have diabetes and 1 out 3 have diabetic retinopathy. It’s important to find out if anyone has diabetic retinopathy early and treat it. If left untreated it eventually leads to blindness. If anyone is diabetic so they can help or prevent or slow the development of diabetic retinopathy by taking prescribed medication, sticking the diet, exercising regularly, controlling high blood pressure or high cholesterol, avoid alcohol and smoking, hence timely diagnosis and regular eye check-up’s along with optimal control of blood sugar through diet, exercise and medication is of most importance to effectively manage diabetic retinopathy
Method applied for Diabetic Retinopathy
The study was approved by the Ethics Committee of Shanghai Sixth People’s Hospital and conducted in accordance with the Declaration of Helsinki. Informed consent was obtained from participants. In the SIM project, retinal photographs were captured using desktop retinal cameras from Canon, Topcon, and ZEISS (Supplementary Table 1). All the fundus cameras were qualified by the organizer to ensure enough quality for DR grading. The operators of the cameras had all received standard training and the images were read by a centered reading group consisting of 133 certified ophthalmologists. The members in the reading group underwent training by fundus specialists and passed the tests. Original retinal images were uploaded to the online platform, and the images of each eye were assigned separately to two authorized ophthalmologists. They labeled the images using an online reading platform and gave the graded diagnosis of DR (Supplementary Fig. 2). The third ophthalmologist who served as the senior supervisor confirmed or corrected when the diagnostic results were contradictory. The final grading result was dependent on the consistency within these three ophthalmologists. At least 20% of the grading results would be randomly re-read to check the consistency. The total eligibility rate of spot-check was equal to or greater than 90%. If the reading group encountered difficult cases, they could apply for consultation from superior medical institutions. The overall disagreement rate in the SIM dataset was 18.9%. The primary cause of the diagnostic divergence was the decision between mild NPDR and non-DR. For retinal lesion annotation, each fundus image was annotated by two ophthalmologists. For each type of lesion, two ophthalmologists generated two lesion annotations, respectively. We considered the two annotations to be valid if the IoU between them was greater than 0.85. Otherwise, a senior supervisor would check the annotations and give feedback to provide guidance. The image would be re-annotated by the two ophthalmologists until the IoU was larger than 0.85. Finally, we took the union of valid annotations as final ground truth segmentation annotation. DR severity was graded into five levels (non-DR, mild NPDR, moderate NPDR, severe NPDR, or PDR, respectively), according to the International Clinical Diabetic Retinopathy Disease Severity Scale (AAO, October 2002)53. Mild NPDR was defined as the presence of microaneurysms only. Moderate NPDR was defined as more than just microaneurysms but less than severe NPDR, presenting CWS, hard exudates, and/or retinal hemorrhages. Severe NPDR was defined as any of the following: more than 20 intraretinal hemorrhages in each of the 4 quadrants; definite venous beading in 2+ quadrants; prominent intraretinal microvascular abnormalities (IRMA) in 1+ quadrant, and no signs of PDR. PDR was defined as one or more of the following: neovascularization, vitreous/preretinal hemorrhage53. DME was diagnosed if hard exudates were detected within 500 μm of the macular center according to the standard of the Early Treatment for Diabetic Retinopathy study54. Referable DR was defined as moderate NPDR or worse, DME, or both. Based on the guidelines for image acquisition and interpretation of diabetic retinopathy screening in China55, the image quality was graded according to standards defined in terms of three quality factors, artifacts, clarity, and field definition56, as listed in Table 5. The total score was equal to the score for clarity plus the score for field definition and minus the score for artifacts. A total score less than 12 was considered as ungradable.
Architecture of the DeepDR system
The DeepDR system had three sub-networks: image quality assessment sub-network, lesion-aware sub-network, and DR grading sub-network. Those sub-networks were developed based on ResNet41 and Mask-RCNN57. Both ResNet and Mask-RCNN could be divided into two parts: (1) feature extractor, which took images as input and output features, (2) task-specific header, which took the features as input and generated task-specific outputs (i.e., classification or segmentation). Specifically, we chose to use the Mask-RCNN and ResNet with the same feature extractor architecture, so the feature extractor of one sub-network can be easily transferred to another. The quality assessment sub-network can identify overall quality including gradability, artifacts, clarity, and field issues for the input images. To train the image quality assessment sub-network effectively, we initialized a ResNet with weights pre-trained on ImageNet and pre-trained the ResNet to form the DR base network. We utilized the weights of the convolution layers in the pre-trained DR base network to initialize the feature extractor of the image quality assessment sub-network. We assessed image quality in terms of multiple factors to determine if: (a) the artifact covered the macular area or the area of artifact was larger than a quadrant of the retinal image; (b) only Level II or wider vascular arch and obvious lesions could be identified (Level II vascular arch was defined as the veins deriving from the first bifurcation); (c) no optic disc or macula was contained in the image; and (d) the image was not gradable. The lesion-aware sub-network can generate lesion presence and lesion segmentation masks of the input images. There were two modules in our lesion-aware sub-network: one was the lesion detection module and the other was the lesion segmentation module. The lesion detection module was a binary classifier that predicted whether any kind of lesions exist in a quadrant of the retinal image, as shown in Supplementary Fig. 3. The lesion segmentation module generated mask images to identify different lesions existing in the retinal images, as shown in Fig. 3B. We used ResNet and Mask-RCNN to form the lesion detection module and lesion segmentation module, respectively. Then we transferred the pre-trained DR base network to the lesion detection module by initializing the feature extractor of lesion detection module using the feature extractor of pre-trained DR base network, followed by fine-tuning the lesion detection module. Then we initialized the feature extractor of lesion segmentation module by reusing the feature extractor of the lesion detection module. The feature extractor layers of the lesion segmentation module were then fixed, and the rest of the layers of the module were updated during training. Non-maximum suppression was used in our lesion segmentation sub-module to select the bounding box with the highest objectiveness score from multiple predicted bounding boxes. Specifically, we first selected the bounding box with the highest objectiveness score, and then compared the IoU of this bounding box with other bounding boxes and removed the bounding boxes with IoU > 0.5. Finally, we moved to the next box with the highest objectiveness score and repeated until all boxes were either removed or selected. The DR grading sub-network can fuse features from lesion-aware network and generate final DR grading results. To retain as much lesion information from the original retinal image as possible, we combined the pre-trained DR base network with the feature extractor of the lesion segmentation module in order to capture more detailed lesion features for DR grading. Then the weights in the extractors of DR grading sub-network were fixed, and the classification header of sub-network was updated during training. The transfer learning assisted multi-task network was developed in our DeepDR architecture to improve the performance of DR grading based on lesion detection and segmentation. Due to the fact that DR grading inherently relies on the global presences of retinal lesions that contain multi-scale local texture and structures, the central feature of our multi-task learning method was designed to extract multi-scale features encoding local textures and structures of retinal lesions, where the transfer learning was used to improve the performance of DR grading task. Meanwhile, we used hard-parameter sharing in lesion-aware sub-network, and all the layers in the feature extractors of ResNet and Mask-RCNN are shared. Using hard-parameter sharing was important to reduce the risk of overfitting58 due to the limited number of lesion segmentation labels. Besides, sharing the pre-trained weights can facilitate the training of both lesion detection task and lesion segmentation task.
Image processing and analysis
As we can see, there is
class imbalance in the training data-set with most cases having value of ‘0’ and least
in ‘1’ and ‘3’ classes.
As we can see, we are trying to spot those hemorrhages/exudates etc. in the higher classes which have DR. To adjust for the images and make more clearer images so as to enable the model to learn features more effectively, we will carry out some image processing techniques using OpenCV library in python (cv2). We can apply Gaussian blur to bring out distinctive features in the images. In Gaussian Blur operation, the image is convolved with a Gaussian filter which is a low-pass filter that removes the high-frequency components. As we can see, we are much more clearly able to see the distinctive patterns in the imgaes now.Here are the image processing applied on 15 image samples.
Model Architecture Used
As we can see below, the Research paper uses a Multi Task learning Model (it parallely does training for Regression, Classification, Ordinal Regression). This way it can use single Model and since first layers would anyway learn similar features, this architecture is implemented to reduce training time (instead of training 3 seperate models). For the Encoder part, we could use any Existing CNN architecture — ResNet50, EfficientNetB4, EfficientNetB5 (and ensemble these).
DEVLOPERS
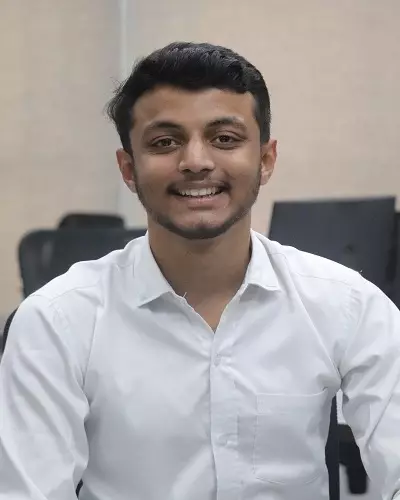
Harsh Viradia
Connect with
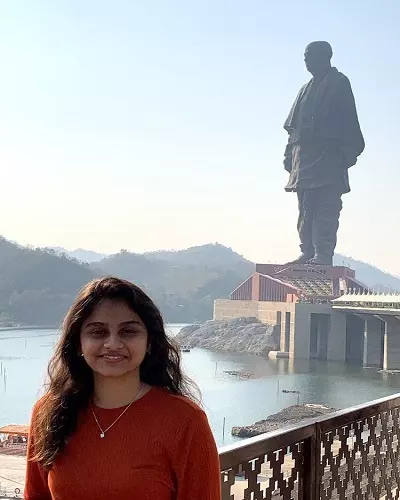